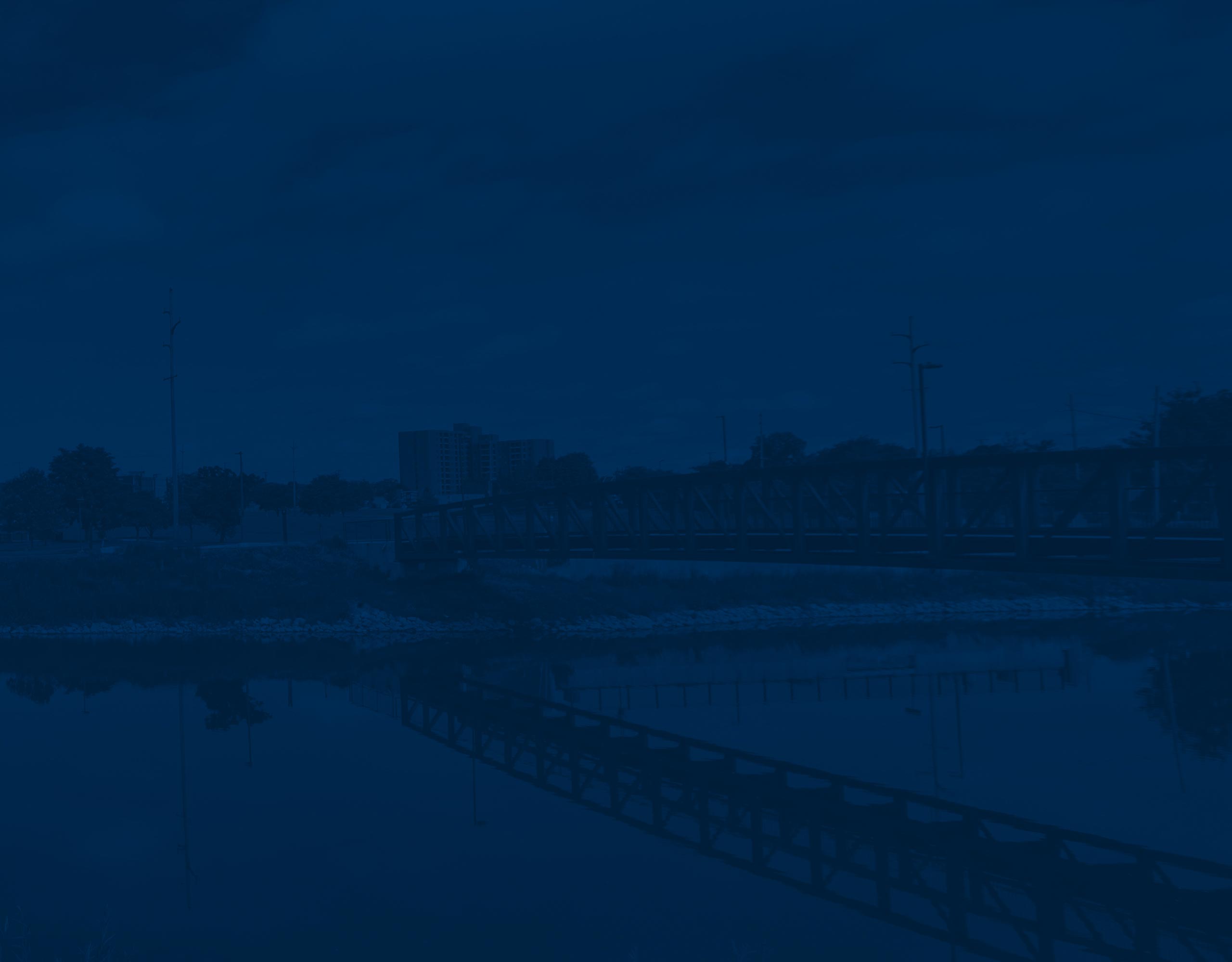
Seeding to Accelerate Research Themes
Seeding To Accelerate Research Themes is a new program in College of Innovation & Technology intended as a way to accelerate preliminary research and collaborative research. START provides a mechanism for faculty members to obtain preliminary results for new and innovative research that is not yet ready for external proposal submission. All tenured or tenure-track assistant/associate/full professors are eligible to receive a token worth up to $15K, propose joint research projects with other CIT colleagues, and then request funds by pooling 2 to 5 tokens to obtain up to $75K. Funds should be used to catalyze bold new research to obtain preliminary results for eventual external proposal submission.
Project Title: Impacts of Tears Size and Location on Blood Flow Dynamics in Type-B Aortic Dissection
Project Description: Aortic dissection is a serious cardiac disease and is considered to be highly lethal, with a mortality rate that increases by 1-2% per hour after symptom onset. Acute dissections are characterized by tissue tears that occur in the inner layer of the aorta. These tears at the inner layer of the aortic wall allow blood to flow under pressure to insinuate itself within the layers of the aortic wall forcing them apart. The two lumens are thus created and separated by a flap tissue that consists of the intima and approximately the inner third of the media wall layer. Alteration in the aortic blood flow behavior due to aortic dissection is not completely understood. Different clinical intervention treatments are currently used to restore normal aortic blood flow dynamics including septectomy and grafting techniques. In this study, we hypothesize that septectomy intervention techniques can be a favorable and efficient treatment of type-B aortic dissections. The treatment can be used to eliminate the pressure differential that exists between the true and false lumen and thus arrest the development of an aneurysm at the site of dissection with fewer risks of complications. Avoiding the persistently higher diastolic pressure in the false lumen can be accomplished by incising the flap. This eliminates the double-barreled flow arrangement that creates a differential of pressure and is likely to contribute to the aneurysmal dilatation.
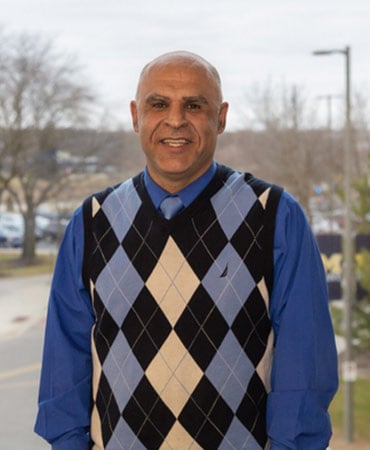
Khalil Khanafer
Assistant Professor
Research: Computational Fluid Dynamics, Additive Manufacturing, Biomechanics, Heat and Mass Transfer, Porous Media, Nanotechnology
810-766-6735
[email protected]
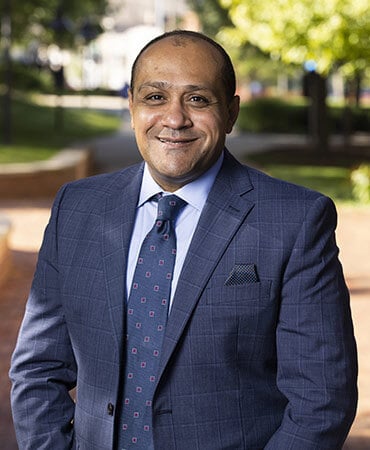
Yasser Aboelkassem, PhD
Assistant Professor
Research: Computational Medicine, Cardiac Electromechanics, Biofluids, Microfluidics, ML-AI in Cardiology
[email protected]
Project Title: Developing Containerized Lab Modules of Machine Learning for Cybersecurity
Project Description: The goal of this project is to develop virtualized lab modules using docker containers on top of the existing Cyber lab exercises from the Naval Postgraduate School (Labtainers) that will make use of various machine learning algorithms for attack vectors and vulnerabilities, such as intrusion detection, malware analysis, spam filters, anomaly detection, etc. Supervised, unsupervised, and semisupervised algorithms and clustering, classification, regression, time-series analysis, and deep learning methodologies will be employed from cybersecurity papers published in journals and conferences with real and synthetic data sets. Each lab module will provide a step-by-step walk-through for the student and end-of-exercise programming exercises. Using current problem formulations from ongoing real research will likely result in student-led submissions to peer-reviewed publications, as both PIs have significant experience in getting students involved in published research. The labs will be shared with other institutions per the target funding agency requirements.
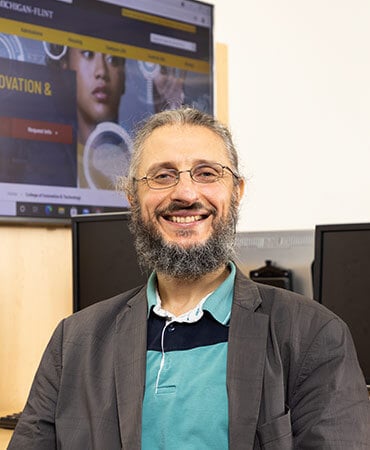
Suleyman Uludag
Professor
810-766-6735
[email protected]
Research: Smart Grid communications, smart meter data collection, security & privacy in the Smart Grid, Cybersecurity education & curriculum development; Routing in Wireless Mesh Networks; Quality-of-Service routing in networks
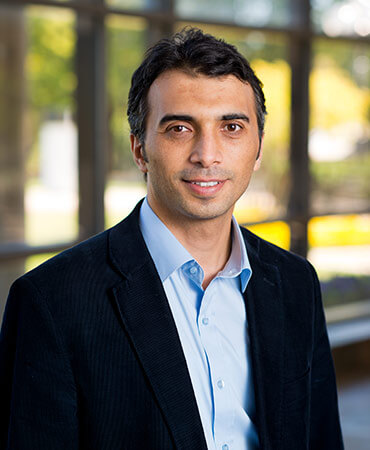
Halil Bisgin
Associate Professor of Computer Science
810-766-6735
[email protected]
Research: Data Mining & Machine Learning, Social Computing, Biomedical Informatics & Bioinformatics, Parallel Computing
Project Title: Augmenting Federated Machine Learning with Sensor Fusion for Autonomous Underwater Robotic Teams
Project Description: Underwater robotic vehicles have emerged as a disruptive technology to support various applications from military concerns to mapping and monitoring marine environments. With increasing levels of autonomy, their impact is further amplified, improving the breath and precision of spacial and temporal measurements. Machine Learning (ML) systems, specifically those which employ deep learning (DL) strategies have gained prominence due to the availability of vast amounts of available data. With this data, we have seen the emergence of systems that outperform humans in many areas. Traditional machine learning approaches as applied to robotics are cumbersome, centralized and mainly occur in isolation Newer methods are moving beyond “siloed” learning, leveraging the computational and sensory capacity of a team, to refine the learning model. Our proposed work seeks to rapidly acquire data through constrained and adaptive foraging in dynamic and obscured environments utilizing a hybrid federated learning approach.
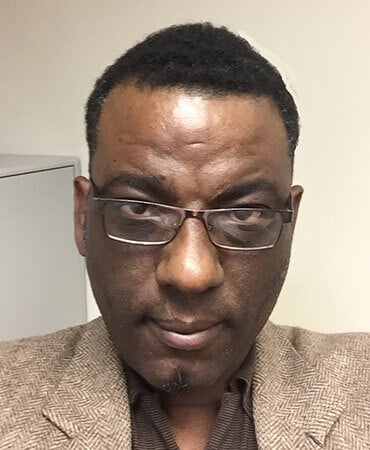
Mark Allison
Associate Professor of Computer Science
Research: Model-Driven Software Development, Smartgrid, Intelligent Agents, UAVs
[email protected]
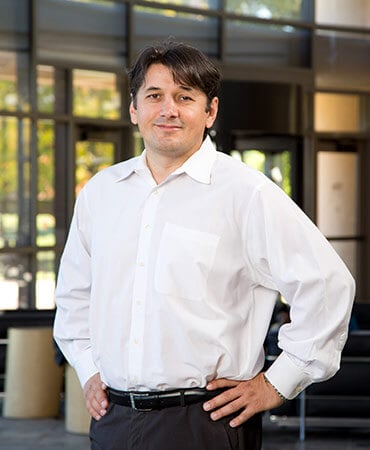
Mihai Burzo
Associate Professor
810-766-6735
[email protected]
Research: Infrared thermal imaging, deception detection, thermal comfort, air diffuser design
Project Title: Towards Meaningful and Real Refactoring Operations
Project Description: Up to 75% of the cost of designing a software system can be attributed to post-deployment maintenance and evolution. In this context, Software Refactoring can lower these expenses by raising the software quality. Software Refactoring has several advantages, but its process can be difficult, error-prone, and time-consuming. Thus, automated refactoring tools have been proposed in the literature aiming to deal with these tasks. However, the state-of-the-art tools still lack trustworthiness when suggesting refactorings. In some cases, the recommended refactorings are inadequate or invalid (e.g., if applied, they can affect the code’s functionality and understanding), leading to developers avoiding such automated tools. Part of this problem is the lack of a comprehensive set of pre-conditions (or rules) used to derive the refactoring recommendation(s). In some cases, these sets of conditions are so simple (or naive) that the refactoring recommendation does not make sense and the developers avoid them and, as a consequence, the automated tools that generate them.
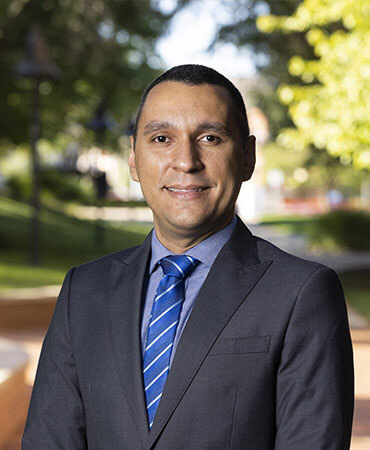
Thiago Ferreira
Assistant Professor
Research: Software Engineering, Search-based Software Engineering, and Evolutionary Optimization Algorithms
[email protected]
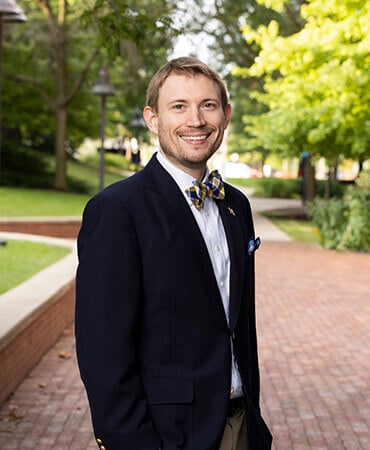
Jeffrey J. Yackley
Assistant Professor
Research: Software Engineering, Software Architecture, Software Evolution and Maintenance, Applied Artificial Intelligence, Game Design, and Computer Science Education
[email protected]
Project Title: Improving productivity via decreasing predictive scrap with Xalt
Project Description: Predictive Scrap
This project seeks to optimize material flow by improving the delivery performance of the vendor base. After analyzing the current value stream map, the research team will create a modeling system that will predict the amount and type of scrap based on orders and materials. The modeling system would collect data to be analyzed and utilized to reduce the inefficiencies in the line. Create a modeling system that would predict the amount and type of scrap based on orders and materials. The modeling system would collect data to be analyzed, and recommend where scrap reduction teams should work next before low-cost scrap materials become larger problems as demand and/or the scrap will increase over time to become a top 10 issue. The modeling system will include the ability to model future output volumes and mixes for employees at Xalt to see the impact and make informed decisions.. There is an employee upskilling component to the initiative included with the simulation.
Upon completion of this project, we can pursue an NSF grant that supports a collaboration such as ours.
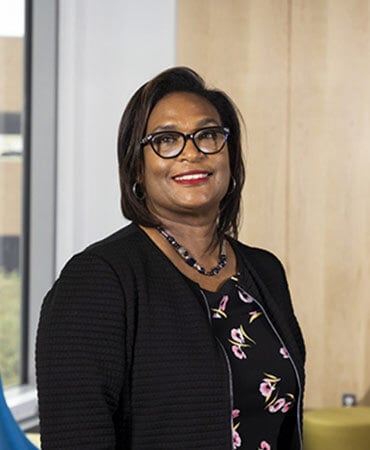
Shirl Donaldson
Assistant Professor
Research: Project Management, Process Improvement, STEM Education, Entrepreneurship
[email protected]
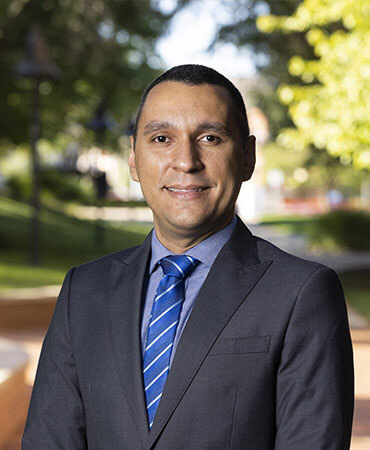
Thiago Ferreira
Assistant Professor
Research: Software Engineering, Search-based Software Engineering, and Evolutionary Optimization Algorithms
[email protected]
Project Title: OpenLabel – Free and open-source news media informational labeling
Project Description: OpenLabel is a free and open-source media labeling Chrome browser extension developed at UM-Flint and designed to work with the Google News feed and Twitter. The extension includes an options menu allowing users to control how the label will be displayed and which categories of information will be included. You can use OpenLabel to become better informed about news articles and their sources before you even open them to read. A working prototype, with features based on user preferences from a representative survey of the US population, is now in the initial phase of testing. Additional features will require developing a deeper repository of data regarding news media sources both local and national which can be easily maintained. Long-term support will require computing resources and funding for such things as web hosting of the backend server as well as advertising of the tool both to consumers and researchers.
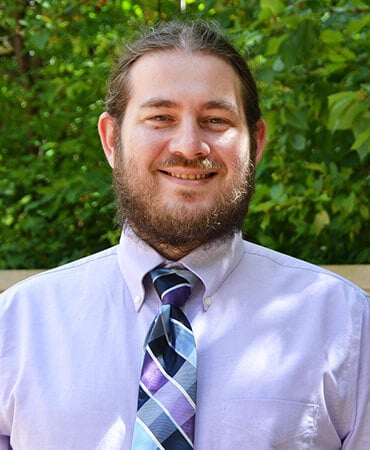
Matthew Spradling
Associate Professor
810-766-6735
[email protected]
Research: Misinformation in Social Media, Coalition Formation, Game Theory, Complexity Theory, Social Networks
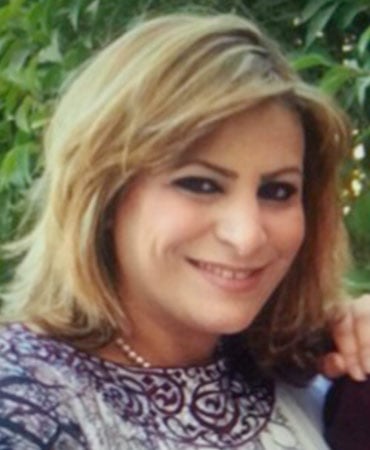
Amal Alhosban
Associate Professor of Computer Science
Research: Semantic Web, Fault Management and Wireless Network
[email protected]
Project Title: Using Consumer-Grade Wearable Devices for Fall Risk Evaluation and Alerts
Project Description: Falls are a major problem prevalent among older adults. It could cause serious injuries that negatively impact their quality of life and can be life-threatening. Evaluating the fall risk for an individual is typically the first step in preventing falls. The risk of falls is commonly evaluated through clinical measurements using rating scales such as Tinetti Performance Oriented Mobility Assessment and Berg Balance Scale. Physical measurements using IMU and force plates are also employed to evaluate an individual’s physical condition as a means to predict the risk of falls. However, these measurements are typically collected by health professionals in clinical settings or research labs, thus making it less accessible to older adults. Therefore, their risk of falling can only be evaluated infrequently. Moreover, changes in their living environment and health conditions would likely entail more frequent assessments. To fill this gap, the development of consumer-grade wearable devices (e.g. smartwatches) has provided the great potential to pervasively collect simple acceleration measurements and the test results can be used for body motion analysis. In the proposed research, we plan to investigate participants’ structured gait features such as TUG (timed up and go) and unstructured gait features (typical walking style and pace in a natural environment) for identifying potential links between wearable measurements and fall risk. While the types and granularity of data on physical activities that can be collected by consumer-grade wearable devices are more limited than using research-grade IMU sensors, we plan to use IMU sensors as benchmarks and evaluate the resolution and accuracy of the data collected via wearable devices. Signal processing algorithms will be employed to extract the critical patterns from wearable device measurements that could be used for daily monitoring. A machine-learning computational model will be developed to correlate the wearable data to clinical scales. We will also explore the design of a mobile app for older adults to self-administer the fall risk test at home. Our app design will be informed by factors such as one’s physical environment, health condition, fear of falls, etc. and the goal is to develop an integrated system that offers fall risk assessment and provides alerts for older adults.
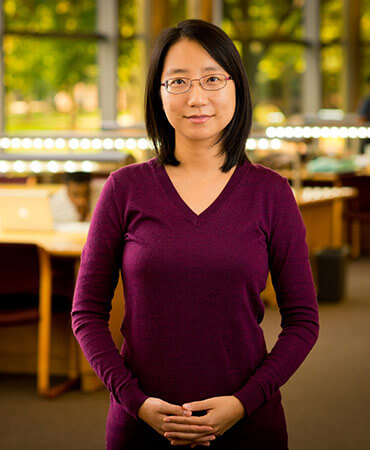
Linda Zhu
Associate Professor of Mechanical Engineering
Research: Acoustics, vibration and noise control
signal processing, sensor, and controls, automotive engineering
[email protected]
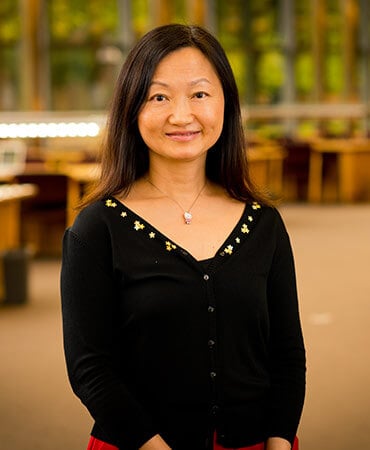
Charlotte Tang
Associate Professor
810-766-6735
[email protected]
Research: Human Computer Interaction, Health Informatics, Computer Supported Cooperative Work, Social Computing, Accessible Computing